題 目:Random forest weighted local manifold estimation
主講人:陳怡璇教授(University of Glasgow)
時 間:113年10月18日(星期五)上午11:10-12:00
地 點:綜合一館427室
使用Google Meet線上直播,
演講開始前20分鐘可進入會議,請點選下列連結後按下「加入」即可
https://meet.google.com/pie-jmyd-cra
摘要
This paper introduces a novel approach for local manifold estimation using random forest-based kernel weights. Traditional methods, such as nonparametric kernel smoothing, often suffer from the curse of dimensionality. In contrast, our method addresses this limitation by implicitly learning forest weights that closely approximate oracle weights. We propose the Gradient Forest Algorithm, which recursively partitions subsamples based on a manifold-adaptive splitting criterion. This process generates a forest of trees, each capturing local geometric structures embedded in the ambient space. By leveraging random subsampling and recursive partitioning, the algorithm produces adaptive neighborhoods that reflect the intrinsic heterogeneity of the data. Our method is particularly well-suited for data with complex geometric properties and high intrinsic dimensionality, where existing local smoothing techniques may struggle. The proposed forest-based ensemble mitigates the high variance associated with individual trees, leading to more stable estimates. This research extends random forest methods by introducing a novel splitting criterion that adapts to the intrinsic properties of data.
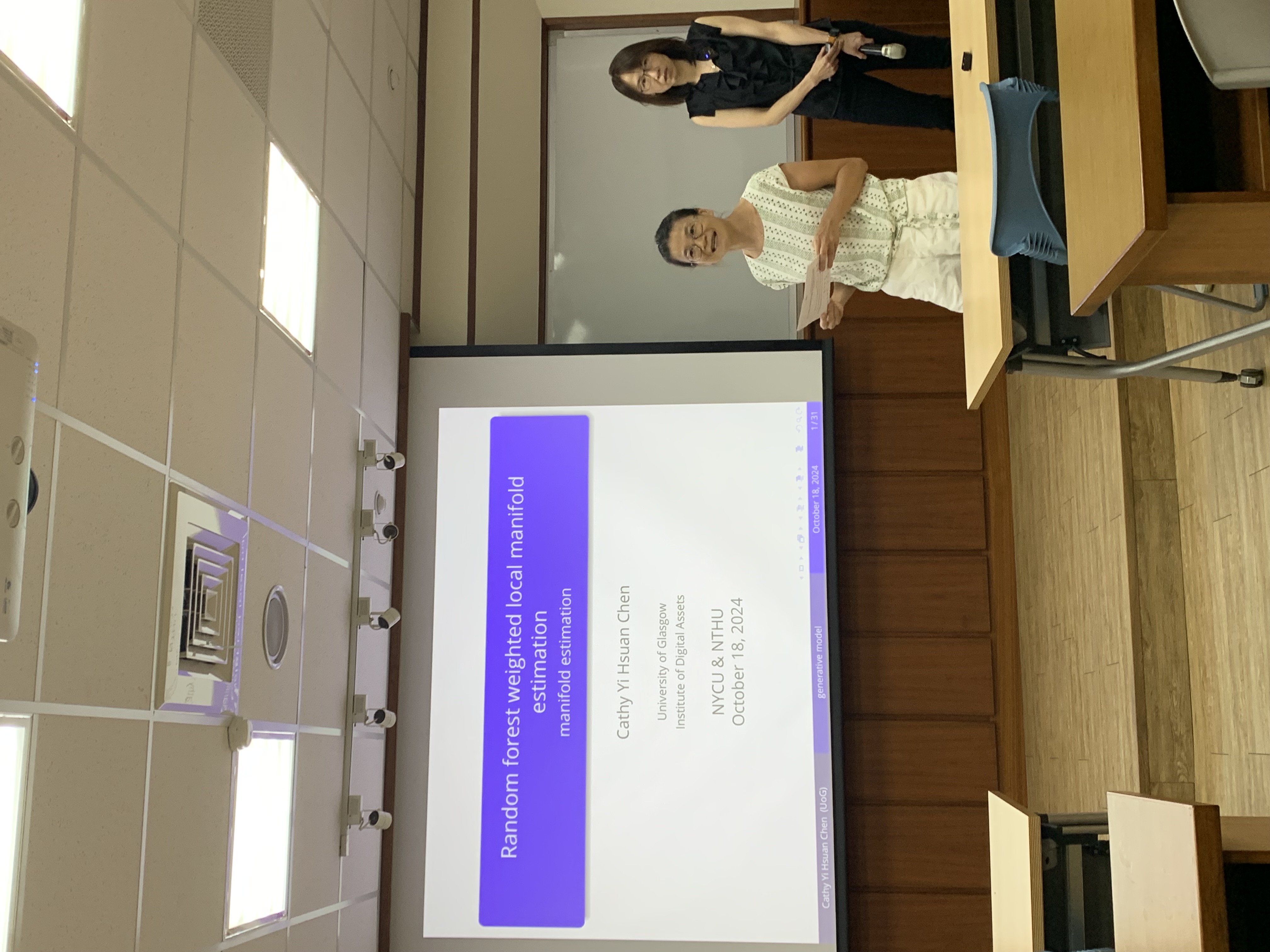
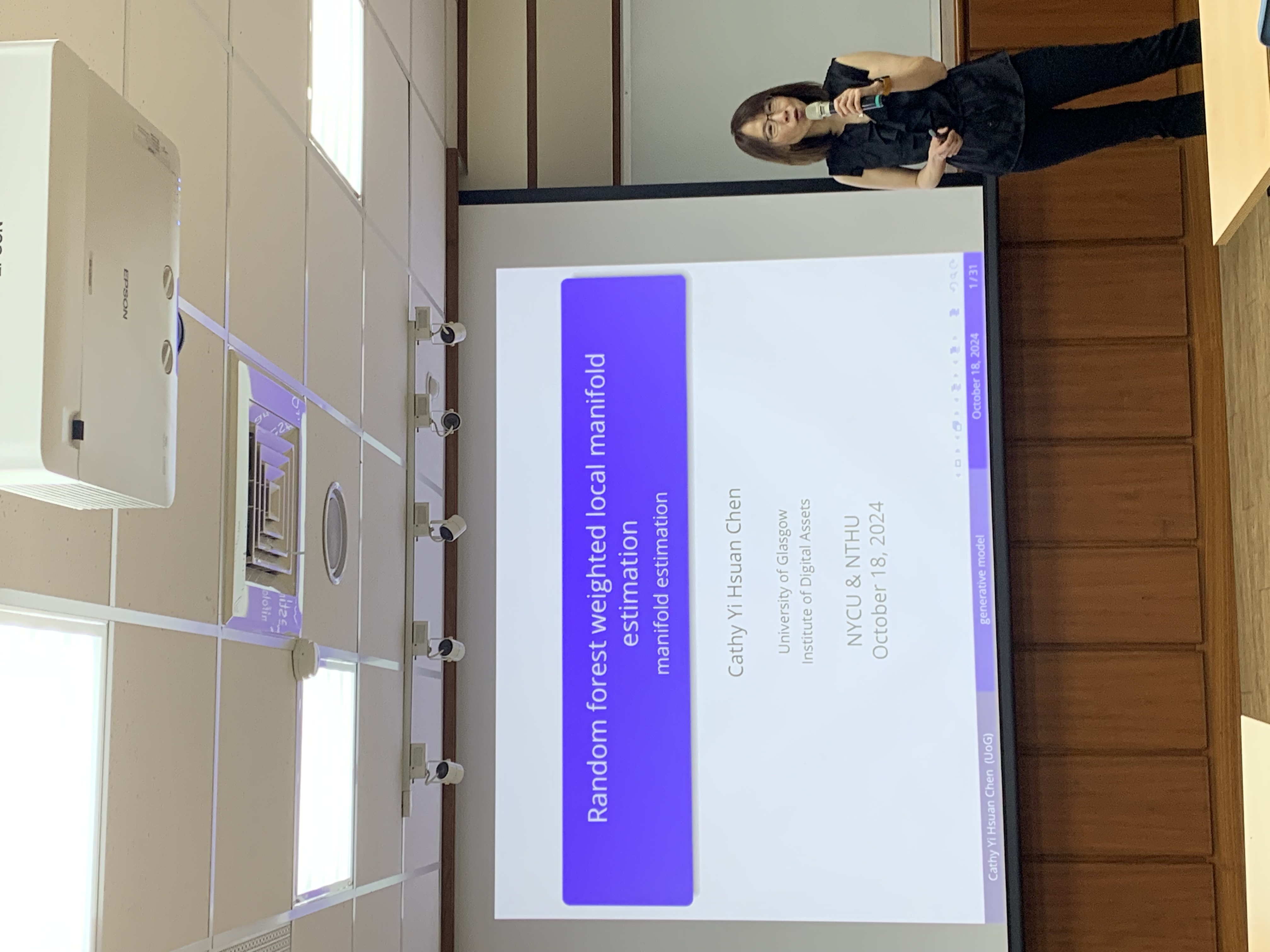