2/16 專題演講 主講人:羅小華教授(哥倫比亞大學統計系教授、陽明交大統計所講座教授)

題 目:Framework for making better predictions by directly estimating variables’ predictivity I, II
主講人:羅小華教授(哥倫比亞大學統計系教授、陽明交大統計所講座教授)
時 間:112年2月16日(星期四)下午14:30-16:30
地 點:綜合一館A427室
摘要
Good prediction, especially in the context of big data, is important. Common approaches to prediction include using a significance-based criterion for evaluating variables to use in models and evaluating variables and models simultaneously for prediction using cross-validation or independent test data. The first approach can lead to choosing less-predictive variables, because significance does not imply predictivity. The second approach can be improved through considering a variable’s predictivity as a parameter to be estimated. The literature currently lacks measures that do this. We suggest a measure that evaluates variables’ abilities to predict, the I-score. The I-score is effective in differentiating between noisy and predictive variables in big data and can be related to a lower bound for the correct prediction rate.
We propose approaching prediction from a framework grounded in the theoretical correct prediction rate of a variable set as a parameter of interest. This framework allows us to define a measure of predictivity that enables assessing variable sets for, preferably high, predictivity. We first define the prediction rate for a variable set and consider, and ultimately reject, the naive estimator, a statistic based on the observed sample data, due to its inflated bias for moderate sample size and its sensitivity to noisy useless variables. We demonstrate that the I-score of the PR method of VS yields a relatively unbiased estimate of a parameter that is not sensitive to noisy variables and is a lower bound to the parameter of interest. Thus, the PR method using the I-score provides an effective approach to selecting highly predictive variables. We offer simulations and an application of the I-score on real data to demonstrate the statistic’s predictive performance on sample data. We conjecture that using the partition retention and I-score can aid in finding variable sets with promising prediction rates; however, further research in the avenue of sample-based measures of predictivity is much desired.
We propose approaching prediction from a framework grounded in the theoretical correct prediction rate of a variable set as a parameter of interest. This framework allows us to define a measure of predictivity that enables assessing variable sets for, preferably high, predictivity. We first define the prediction rate for a variable set and consider, and ultimately reject, the naive estimator, a statistic based on the observed sample data, due to its inflated bias for moderate sample size and its sensitivity to noisy useless variables. We demonstrate that the I-score of the PR method of VS yields a relatively unbiased estimate of a parameter that is not sensitive to noisy variables and is a lower bound to the parameter of interest. Thus, the PR method using the I-score provides an effective approach to selecting highly predictive variables. We offer simulations and an application of the I-score on real data to demonstrate the statistic’s predictive performance on sample data. We conjecture that using the partition retention and I-score can aid in finding variable sets with promising prediction rates; however, further research in the avenue of sample-based measures of predictivity is much desired.
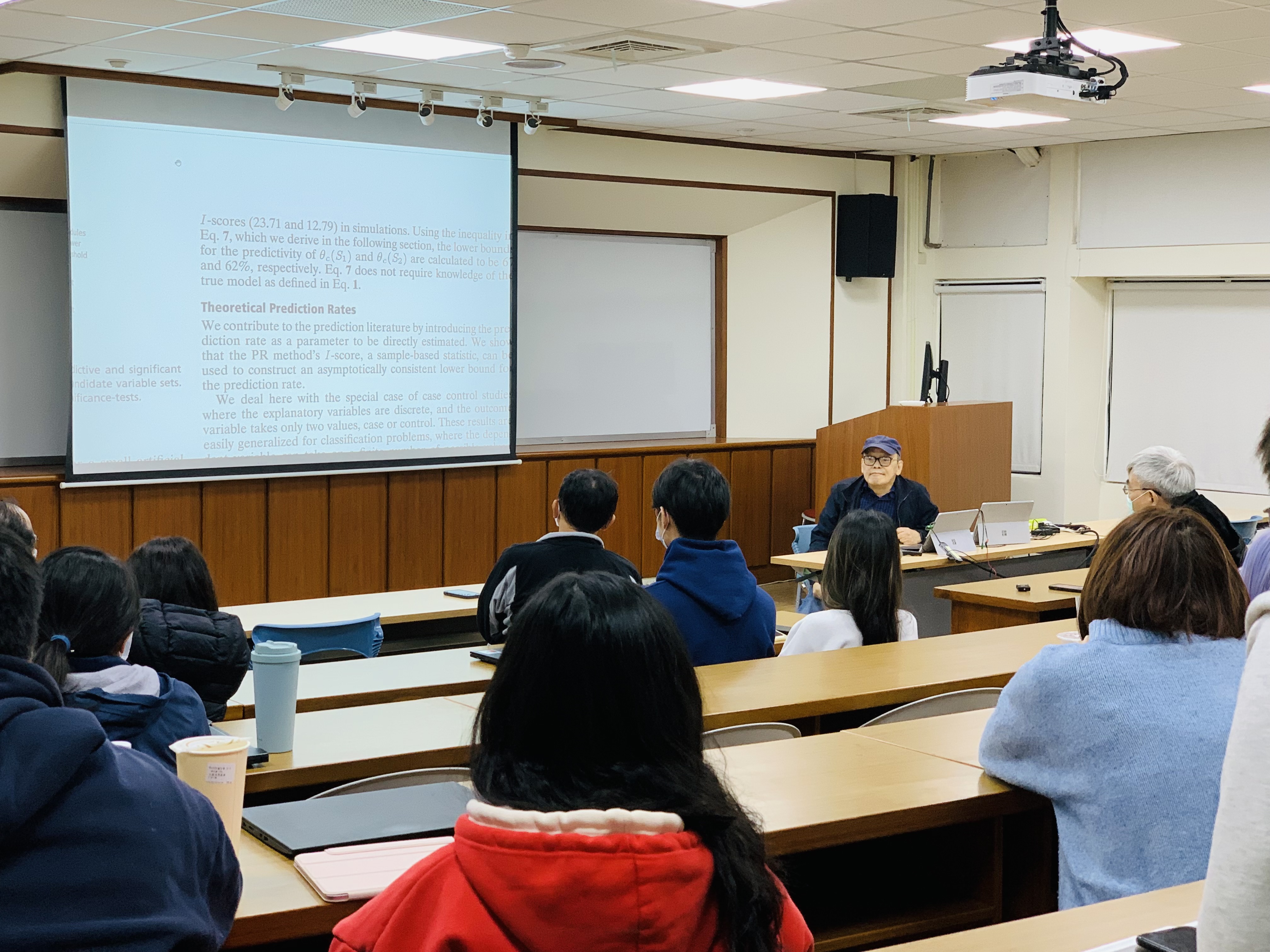